Notice
Recent Posts
Recent Comments
Link
일 | 월 | 화 | 수 | 목 | 금 | 토 |
---|---|---|---|---|---|---|
1 | 2 | 3 | 4 | 5 | 6 | 7 |
8 | 9 | 10 | 11 | 12 | 13 | 14 |
15 | 16 | 17 | 18 | 19 | 20 | 21 |
22 | 23 | 24 | 25 | 26 | 27 | 28 |
29 | 30 |
Tags
- DP
- C++
- LSTM
- 그래프 이론
- image processing
- dfs
- AlexNet
- Mask Processing
- eecs 498
- opencv
- One-Stage Detector
- ubuntu
- real-time object detection
- NLP
- deep learning
- MinHeap
- 강화학습
- Reinforcement Learning
- 백준
- CNN
- r-cnn
- dynamic programming
- BFS
- YoLO
- two-stage detector
- MySQL
- 딥러닝
- machine learning
- 머신러닝
- Python
Archives
- Today
- Total
목록glimpses (1)
JINWOOJUNG
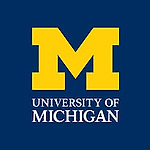
본 포스팅은 Michigan Univ.의 EECS 498 강의를 수강하면서 공부한 내용을 정리하는 포스팅입니다.Introduction 기존까지의 Neural Networks를 활용한 Task는 Image 기반의 Calssification, Detection, Segmentation이 주를 이뤘다. 이러한 Feedforward Neural Network는 1개의 Image를 Input으로 하여, 해당 Image의 Class, Localization 정보 등 1개의 Output을 반환하는 구조이다. 앞으로 살펴볼 Recurrent Neural Network(RNN)은 시계열 혹은 순차 데이터를 처리하고 예측하는 딥러닝을 위한 신경망 구조이다. RNN은 기존의 one to one 구조가 아닌 여러 구조에..
딥러닝/Michigan EECS 498
2025. 2. 8. 17:24