Notice
Recent Posts
Recent Comments
Link
일 | 월 | 화 | 수 | 목 | 금 | 토 |
---|---|---|---|---|---|---|
1 | ||||||
2 | 3 | 4 | 5 | 6 | 7 | 8 |
9 | 10 | 11 | 12 | 13 | 14 | 15 |
16 | 17 | 18 | 19 | 20 | 21 | 22 |
23 | 24 | 25 | 26 | 27 | 28 |
Tags
- object detection
- DP
- One-Stage Detector
- 머신러닝
- two-stage detector
- C++
- dfs
- 그래프 이론
- YoLO
- LSTM
- AlexNet
- 백준
- Python
- CNN
- Mask Processing
- opencv
- r-cnn
- Reinforcement Learning
- BFS
- canny edge detection
- 강화학습
- deep learning
- 딥러닝
- real-time object detection
- eecs 498
- machine learning
- MinHeap
- image processing
- dynamic programming
- MySQL
Archives
- Today
- Total
목록grid cell (1)
JINWOOJUNG
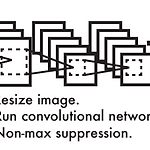
Research PaperYOLO AbstractYOLO는 Object Detection Task의 새로운 접근 방식이다. Object Detection Task를 하나의 Regression Problem으로 정의하며, 하나의 Network로 구현되어 End-to-End 최적화가 가능하다. One-stage Detector인 YOLO는 45fps의 실시간성을 보인다. YOLO는 더 많은 Loclization Error를 보이지만, 배경에 대한 FP를 줄이며, 객체에 대한 일반적인 특징을 잘 학습한다. Introduction Object Detection Task에서 좋은 성능을 보이는 Model은 Classifier를 Detection으로 변형한 구조를 가진다. 특히, R-CNN의 경우 Region Pr..
딥러닝/논문
2025. 1. 19. 00:41