일 | 월 | 화 | 수 | 목 | 금 | 토 |
---|---|---|---|---|---|---|
1 | ||||||
2 | 3 | 4 | 5 | 6 | 7 | 8 |
9 | 10 | 11 | 12 | 13 | 14 | 15 |
16 | 17 | 18 | 19 | 20 | 21 | 22 |
23 | 24 | 25 | 26 | 27 | 28 |
- 딥러닝
- eecs 498
- image processing
- DP
- 백준
- C++
- 머신러닝
- Python
- dynamic programming
- Reinforcement Learning
- deep learning
- real-time object detection
- YoLO
- MinHeap
- AlexNet
- 그래프 이론
- object detection
- CNN
- two-stage detector
- LSTM
- dfs
- machine learning
- One-Stage Detector
- 강화학습
- r-cnn
- MySQL
- opencv
- Mask Processing
- BFS
- canny edge detection
- Today
- Total
목록voxelnet (2)
JINWOOJUNG
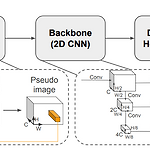
PapaerPointPillars Introduction 자율주행차는 차량, 보행자, Cyclist와 같은 동적 객체를 검출해야한다. LiDAR 3D PointCloud Data(PCD)를 기반으로 한 Object Detection Task는 Bottom-up Pipeline을 통해 연구되어왔다. 즉, Hand-crafted Feature를 기반으로 지면 제거 후 객체에 대한 Clustering, Classification이 진행된다. 단순히 Hand-crafted Feature만으론 한계가 있기 때문에 Computer Vision 분야의 Deep Learning Methods가 도입되면서 PCD에 적용하려고 했지만, Sparse하고 3D 구조인 PCD의 특성 상 2D Image에 적용되는 Convol..
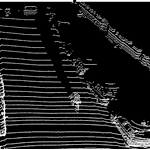
PapaerVoxelNet AbstractSparse한 LiDAR PCD를 Region Proposal Network(RPN)과 연결하는 연구는 Hand-crafted Feature를 기반으로 연구되었다. 본 논문에서는 Feature Extraction과 Bounding Box Prediction을 하나의 Stage로 통합하여 E2E 학습이 가능한 Deep Network인 VexelNet을 제안한다. VoxelNet은 Point Cloud를 동일한 크기의 3D Voxel로 나눈 뒤, 각 Voxel 내 Point를 Voxel Feature Encoding(VFE) Layer를 통해 통합된 특징으로 표현한 결과를 RPN으로 전달하는 구조이다. VoxelNet은 LiDAR Data만 활용하여 Car, Ped..